DAR.10/
DAR.11/
REVISIONING TAIPEI
/ 2205
A New Era in Real Estate Valuation with Sustainability Considerations
Real estate valuation is crucial in numerous sectors, underpinned by models like the Hedonic Price Model, Automated Valuation Models, and Computer-Assisted Mass Appraisal. Traditionally, these models have focused on structural attributes and locational amenities, often overlooking visual features. This project aims to revolutionize this approach by integrating machine learning and sustainability factors into the conventional framework of real estate valuation.
Taipei, chosen for this case study, presents a unique set of market conditions. Ranking as the world's second most unaffordable city and the third most densely populated, Taipei's real estate market is characterized by high demand, steep housing prices, and volatility. Given the limitations of traditional approaches in such dynamic and challenging market scenarios, this environment underscores the need for a more dependable valuation method.
Taipei, chosen for this case study, presents a unique set of market conditions. Ranking as the world's second most unaffordable city and the third most densely populated, Taipei's real estate market is characterized by high demand, steep housing prices, and volatility. Given the limitations of traditional approaches in such dynamic and challenging market scenarios, this environment underscores the need for a more dependable valuation method.
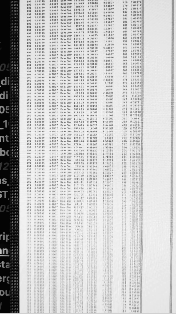
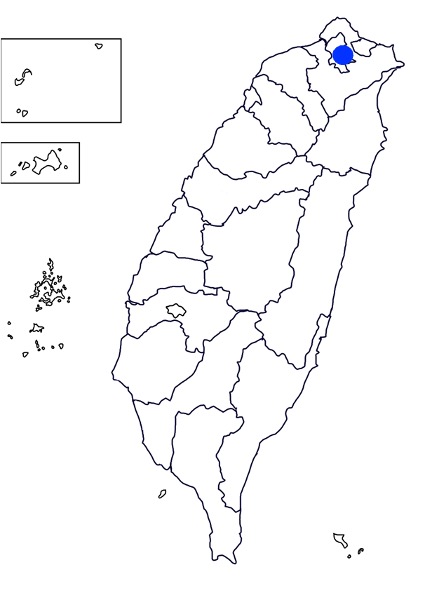
Methodology
This project's methodology encompasses the creation of a multifaceted dataset that serves as the foundation for a machine learning model, integrating housing characteristics, locational benefits, and buyer demographics. It incorporates a variety of socioeconomic variables, the air quality index, and visual inputs from Google Street View to formulate district-level insights. Employing machine learning regression and decision trees, the project endeavors to calculate housing prices, presenting a comparative analysis with traditional valuation methodologies. The Actual Price Registration data provided by the Ministry of Interior, detailing a comprehensive record of real estate transactions from 2012 to 2022, constitutes the core of this dataset. This data is enriched with key socioeconomic elements, including population dynamics, transaction volumes, and housing license and permit statistics, thereby presenting a more complete depiction of the housing market in Taipei.
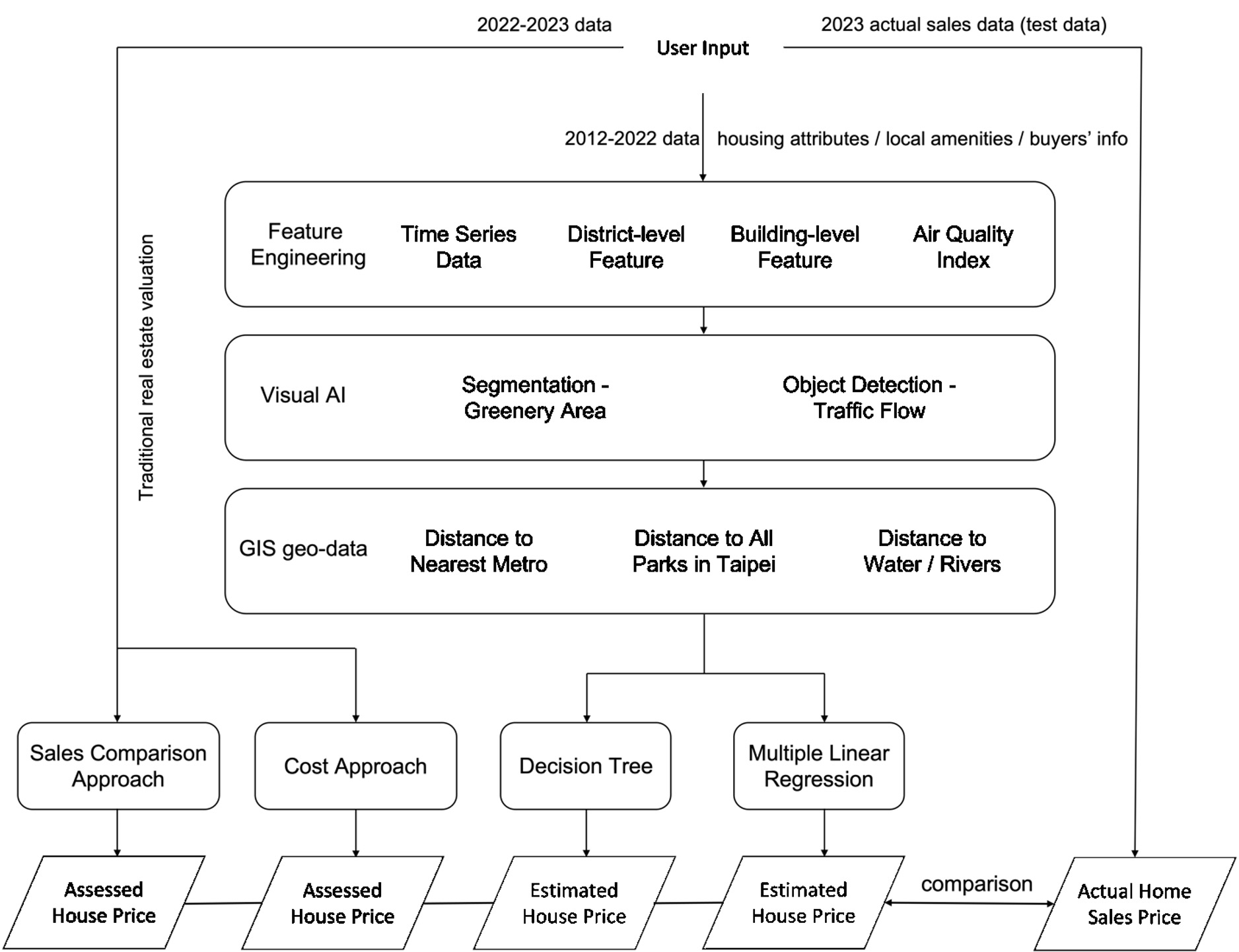
Study Workflow
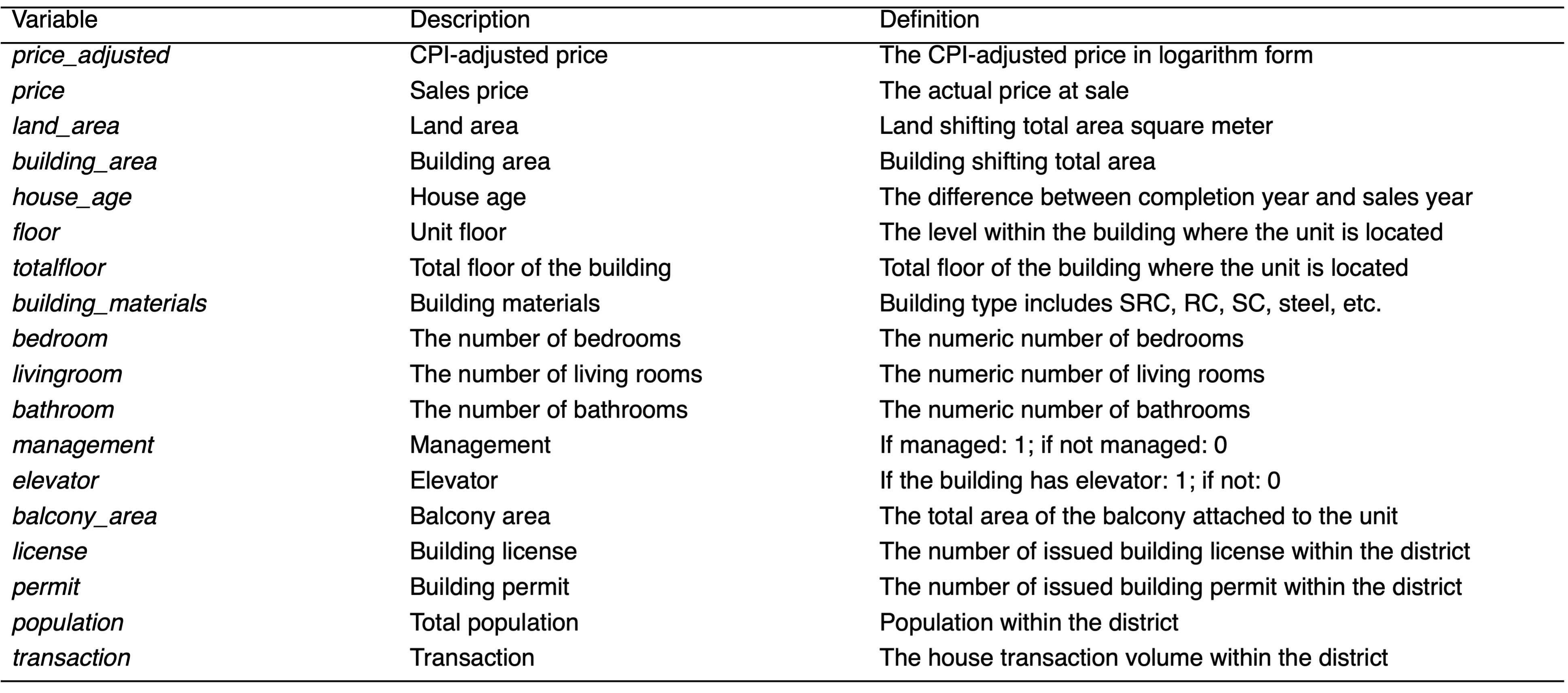
Socioeconomic Factors
Air Quality Index (AQI):
The research incorporates pollutant data from Taiwan's Environmental Protection Administration Executive Yuan, which includes PM2.5, CH4, NO2, NO, NOx, PM10, O3, CO, and SO2, to improve the accuracy of the analysis.
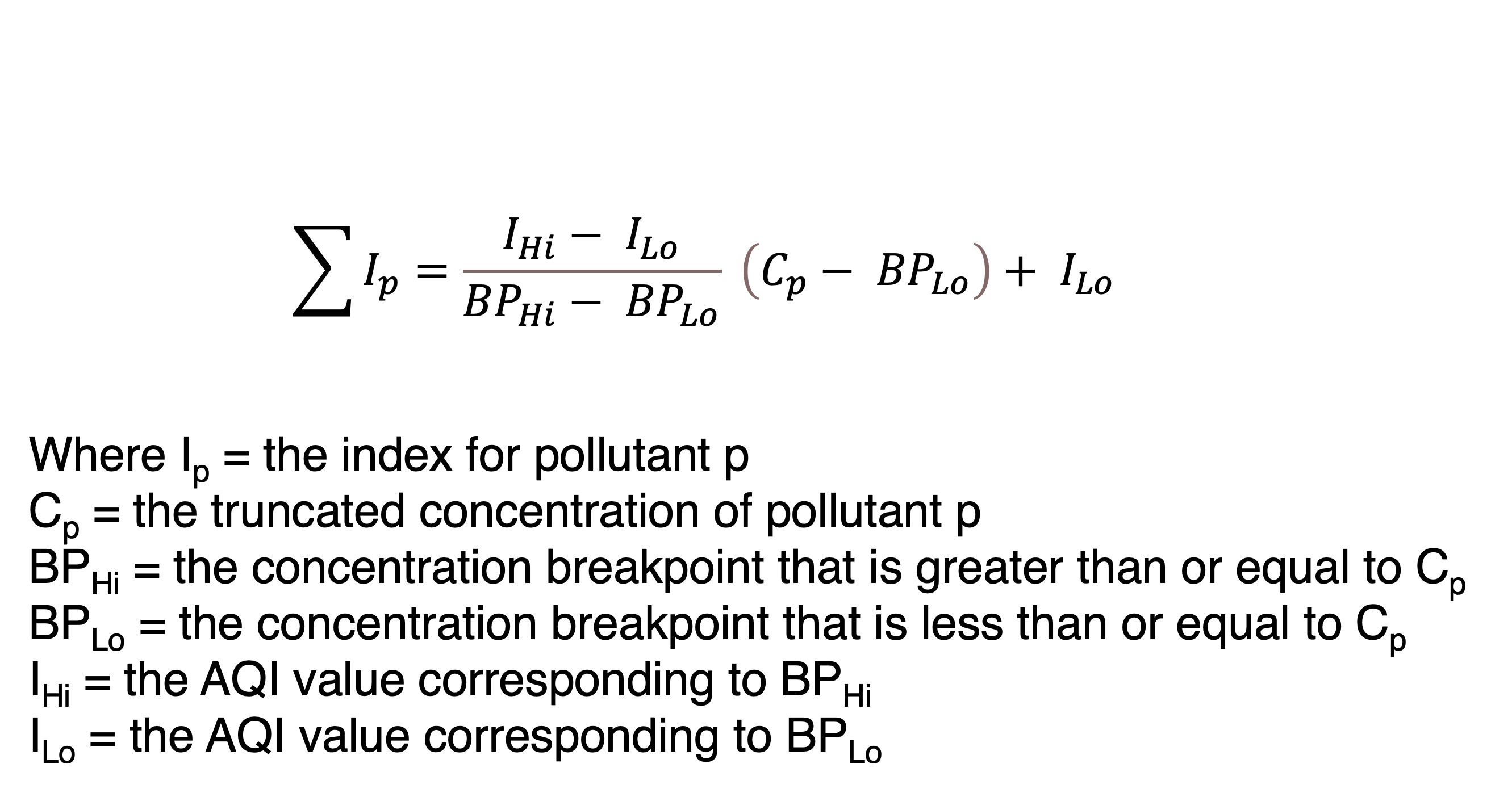
Visual AI Features
Taipei’s Google Street View image segmentation
Visual AI Features
Taipei’s Google Street View image segmentation
Taipei’s Google Street View image segmentation
The project elevates the precision of housing price assessment by incorporating visual AI features through the segmentation of over 110,000 Google Street View images of Taipei. This analysis assesses critical urban elements such as green spaces, traffic levels, and mobility within the city. Although these images represent a specific moment in time, they significantly enhance the machine learning model by providing a visual dimension to the urban landscape data.
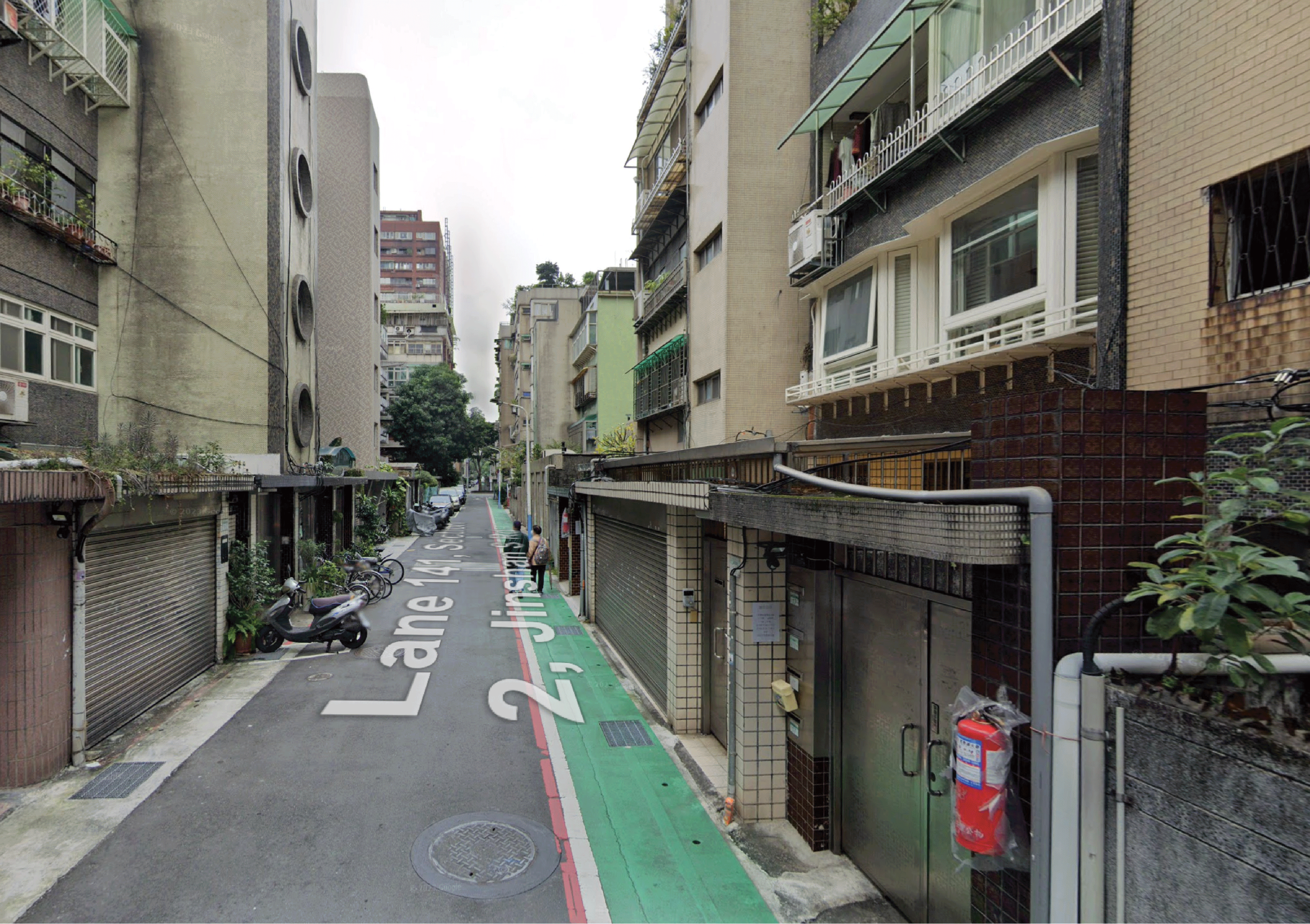
key attributes:
plants, grass, palmtree, tree, car, bus, truck, motorbike, bicycle, sidewalk
key attributes:
plants, grass, palmtree, tree, car, bus, truck, motorbike, bicycle, sidewalk
plants, grass, palmtree, tree, car, bus, truck, motorbike, bicycle, sidewalk
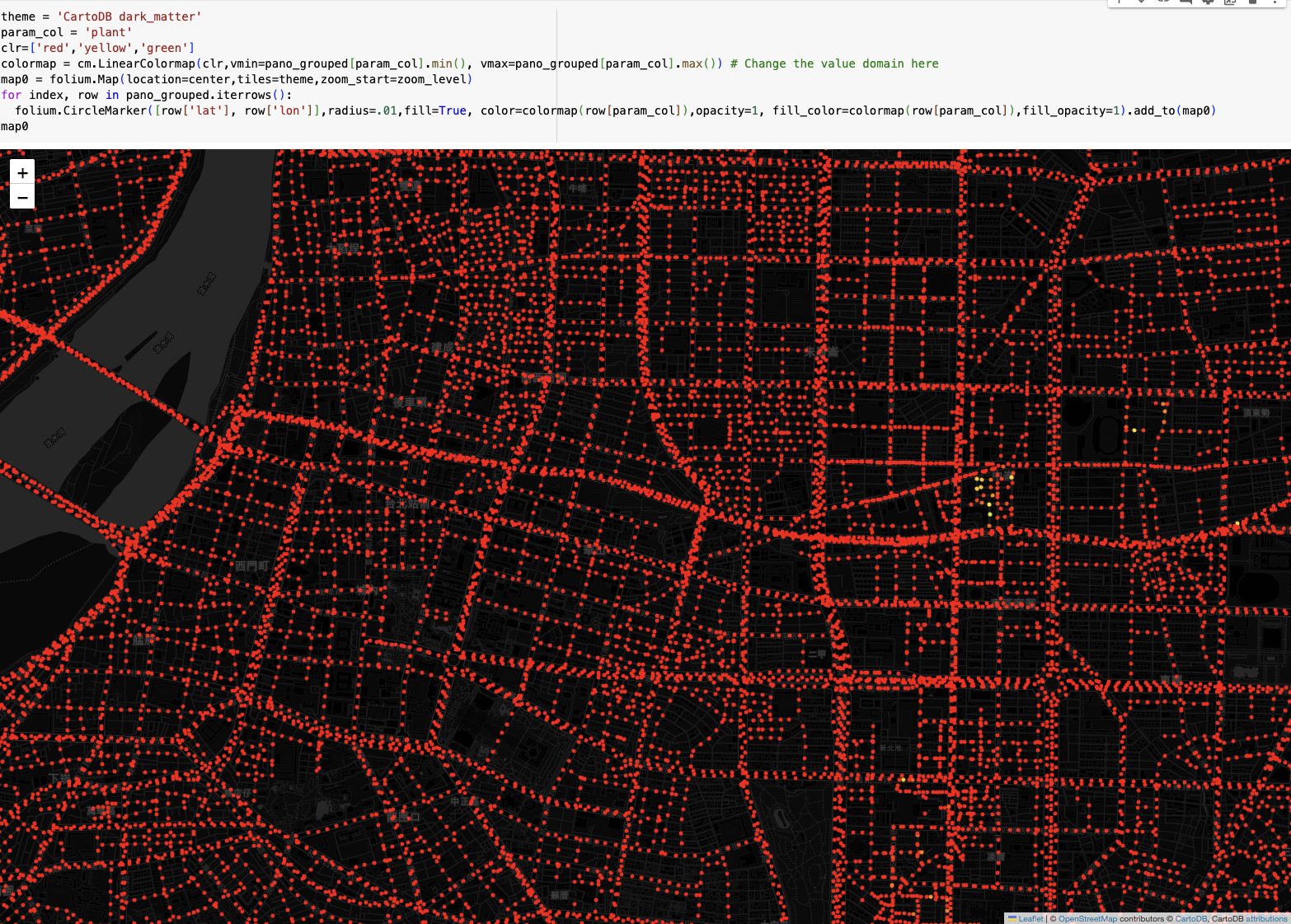

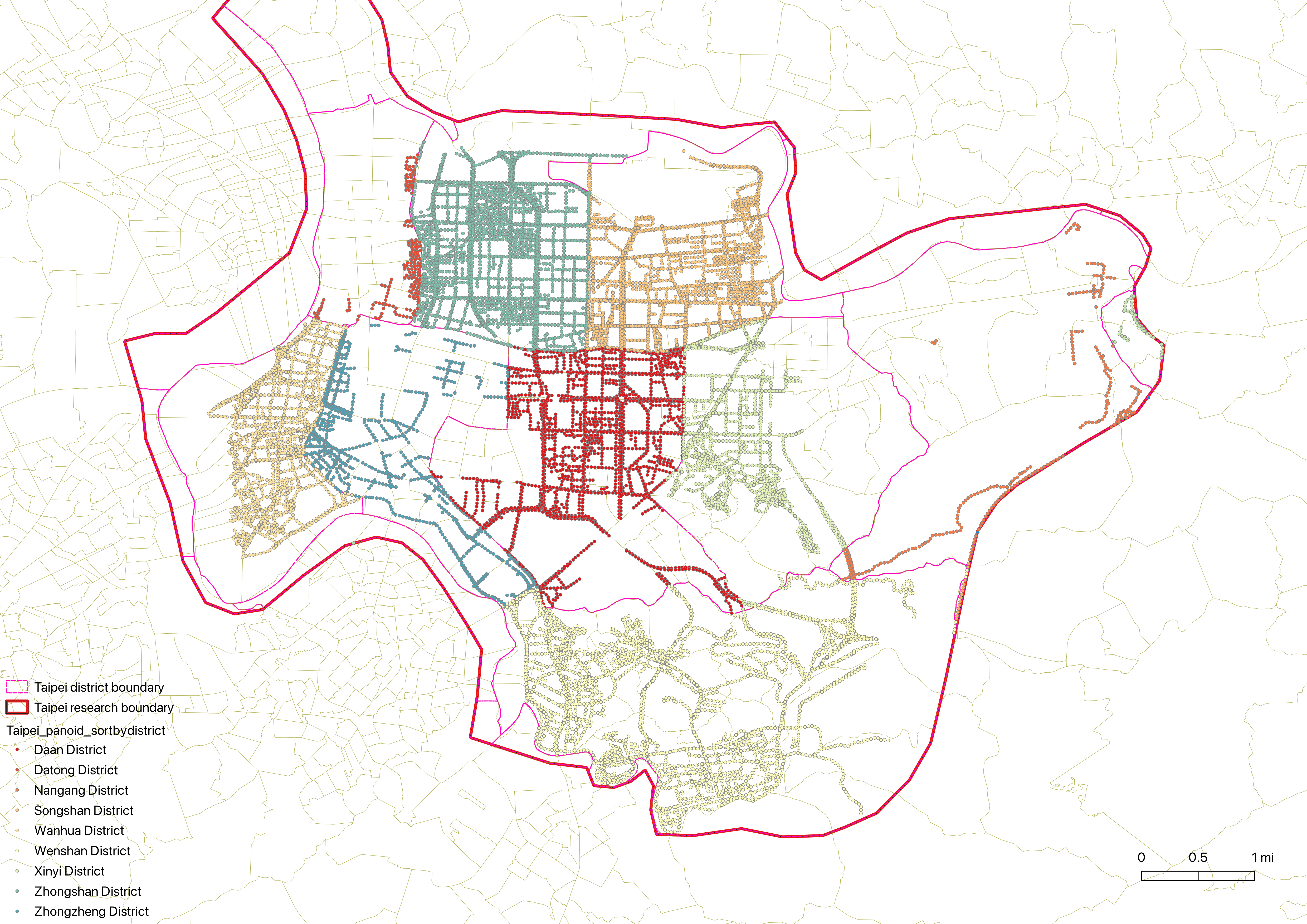
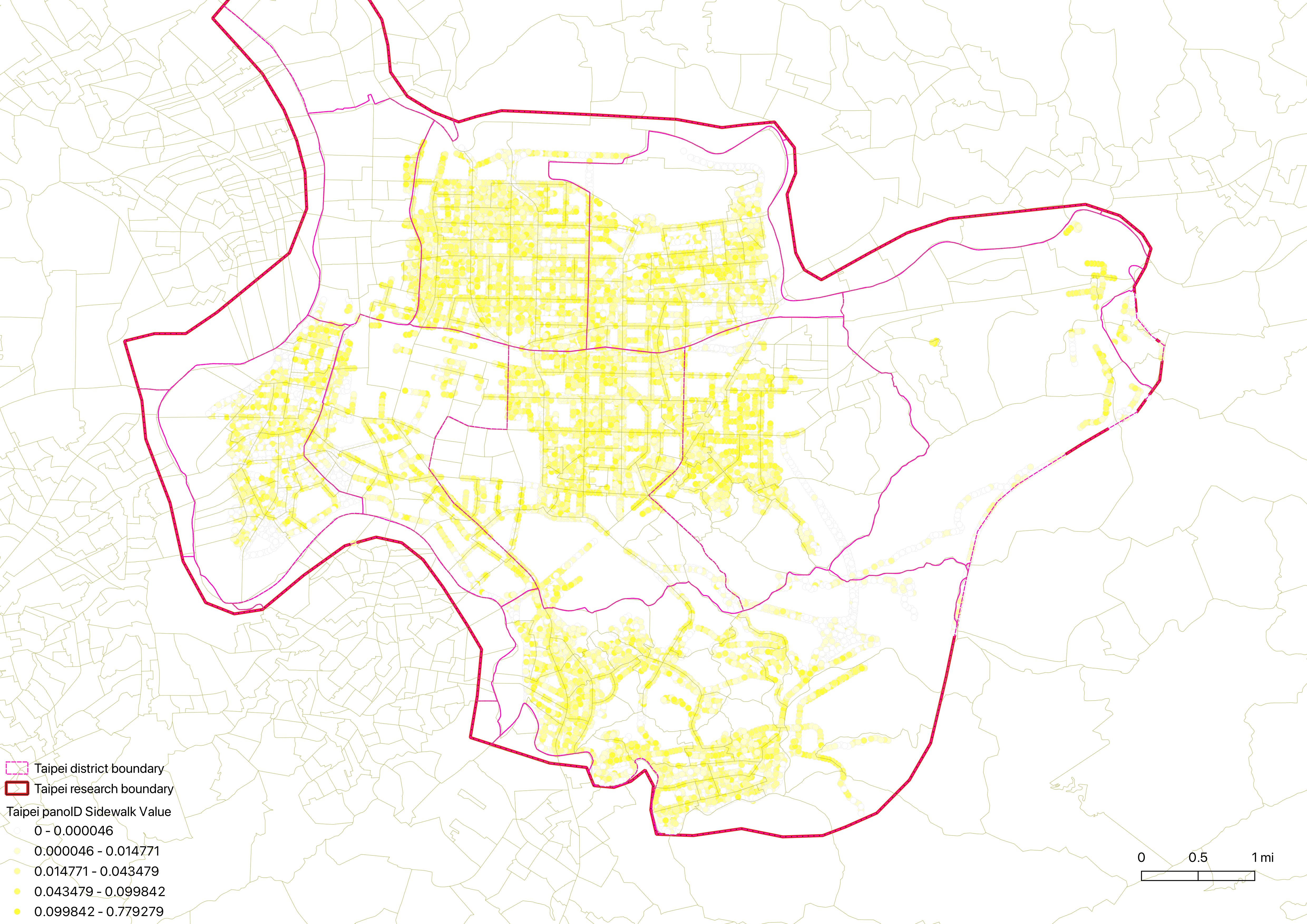
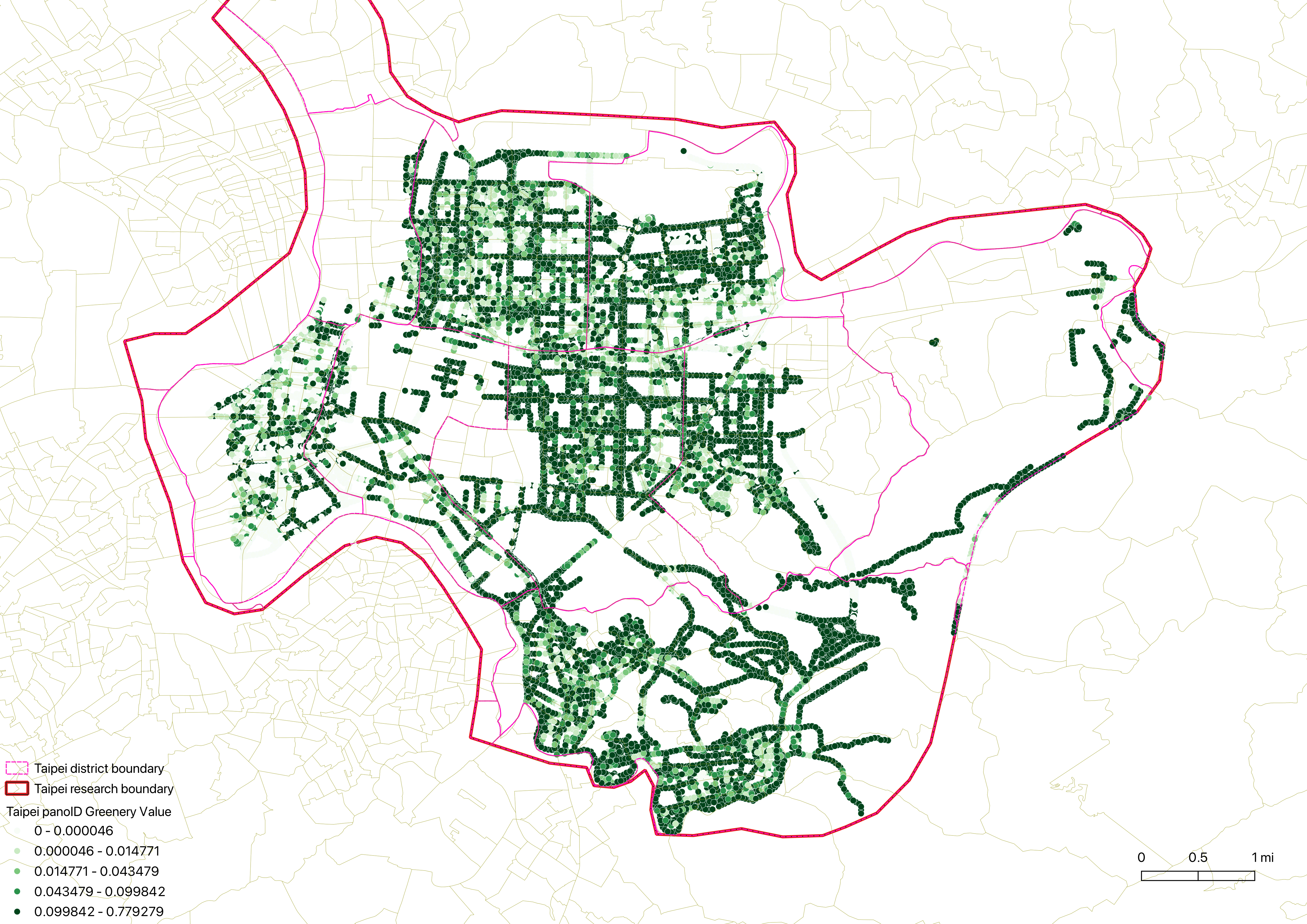
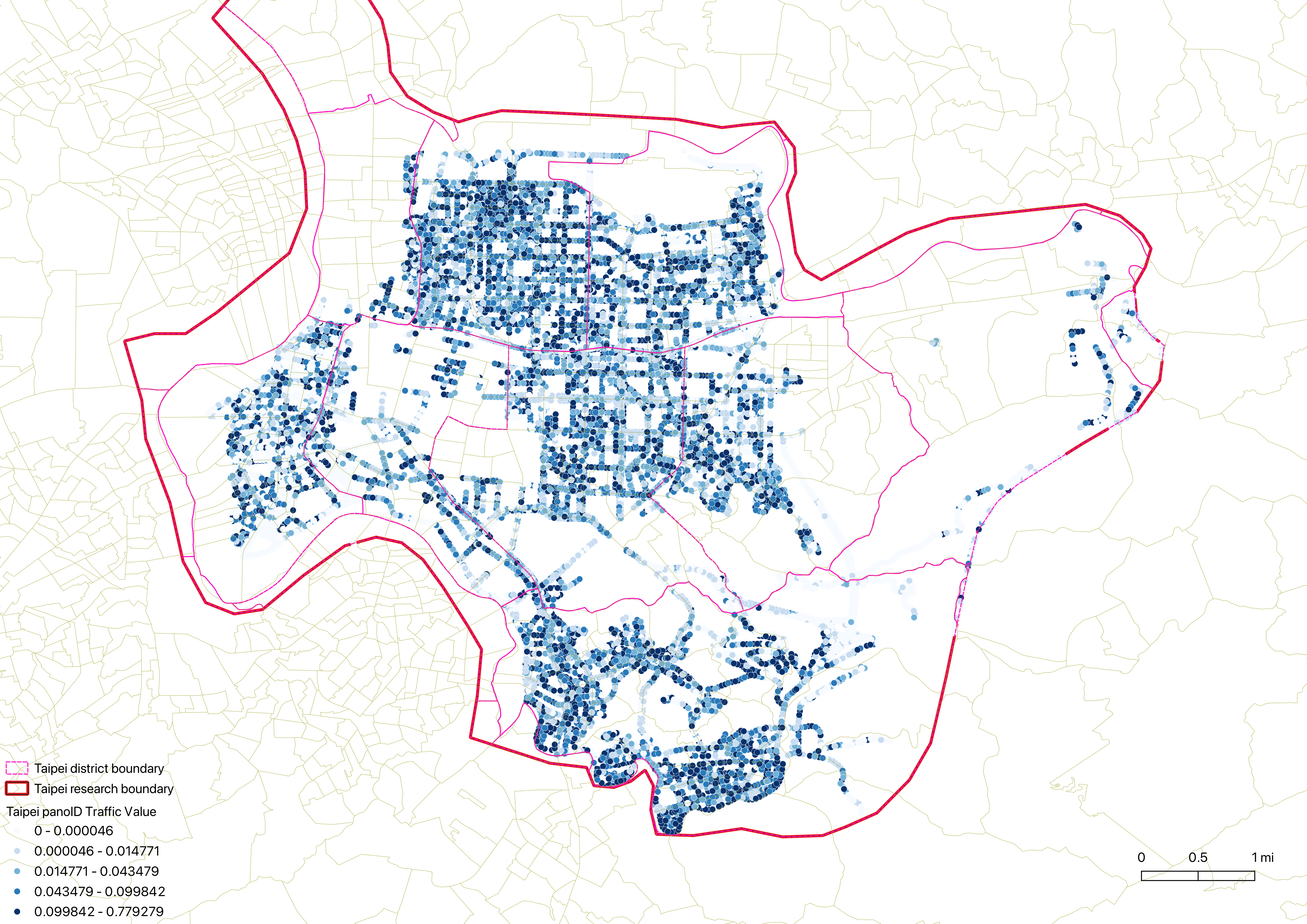
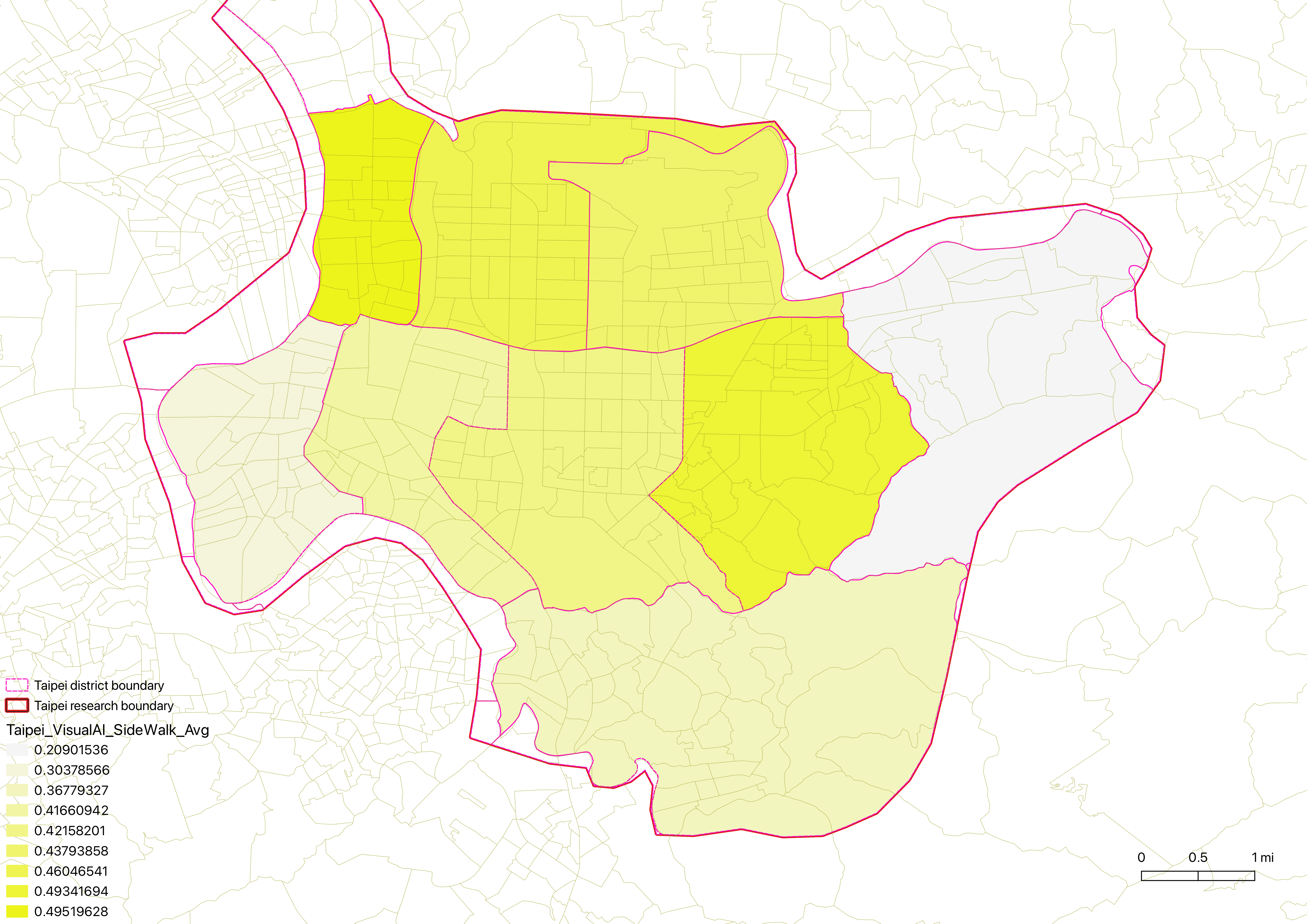
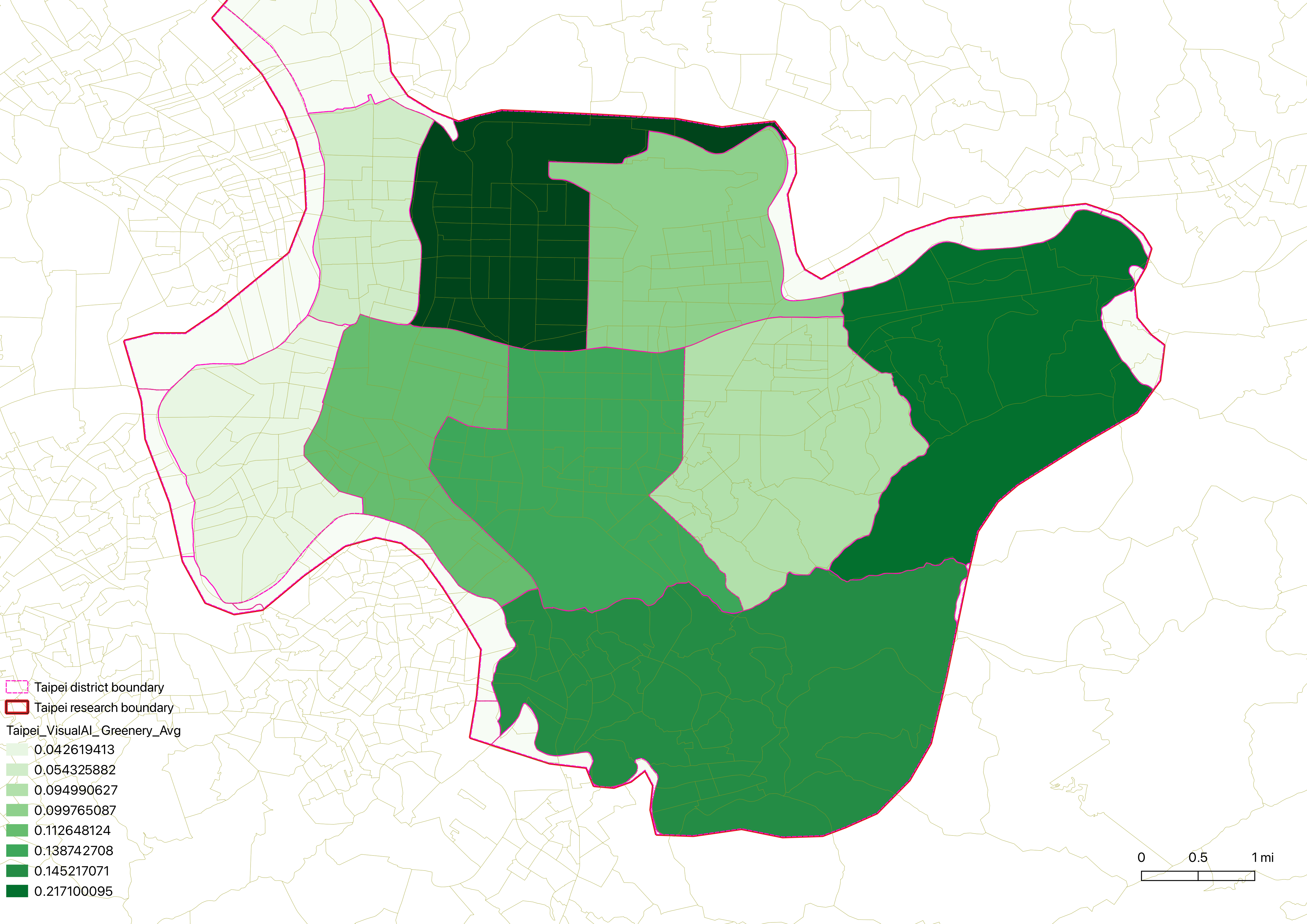
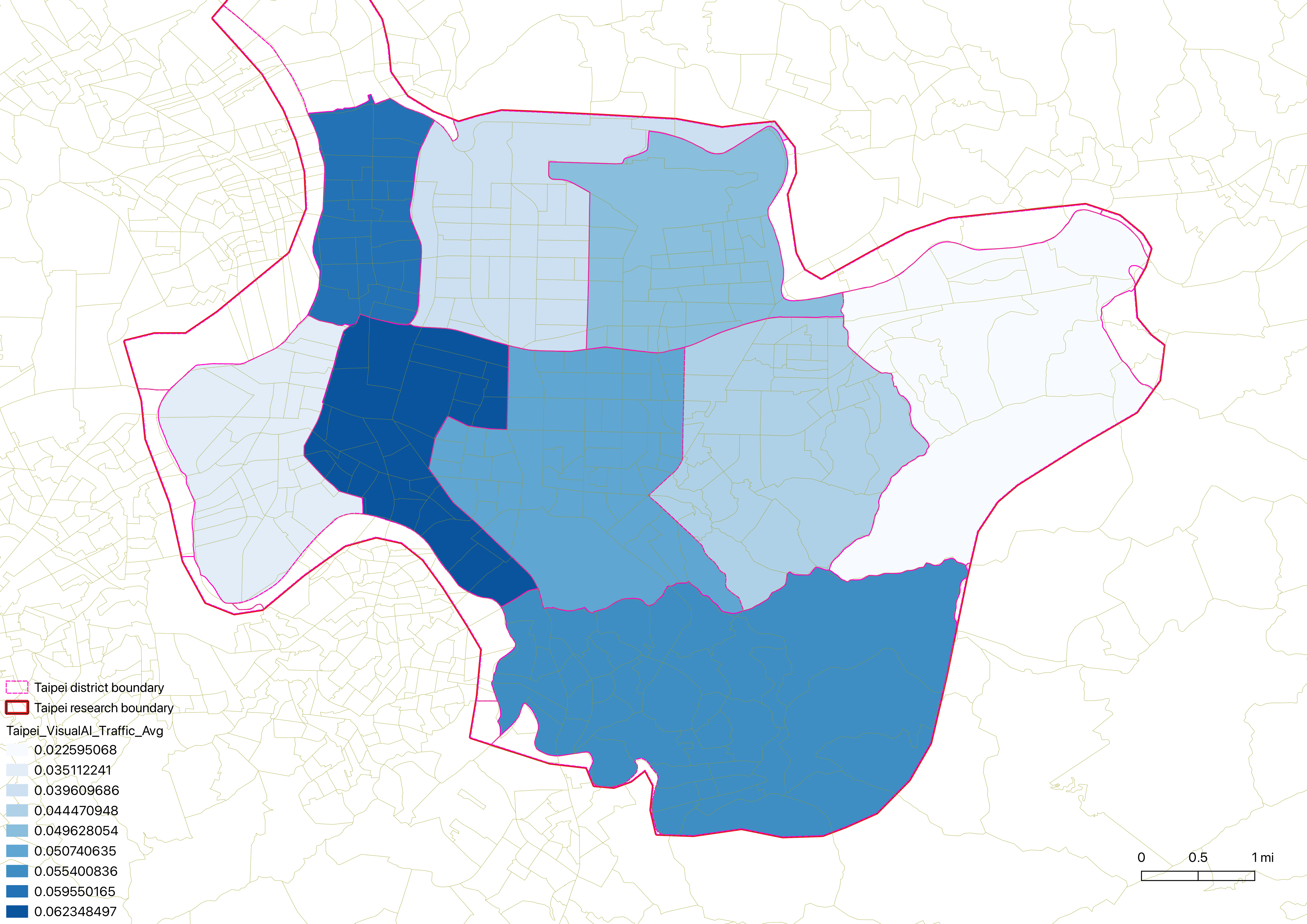
Geospatial Features
Distance to Green Infrastructure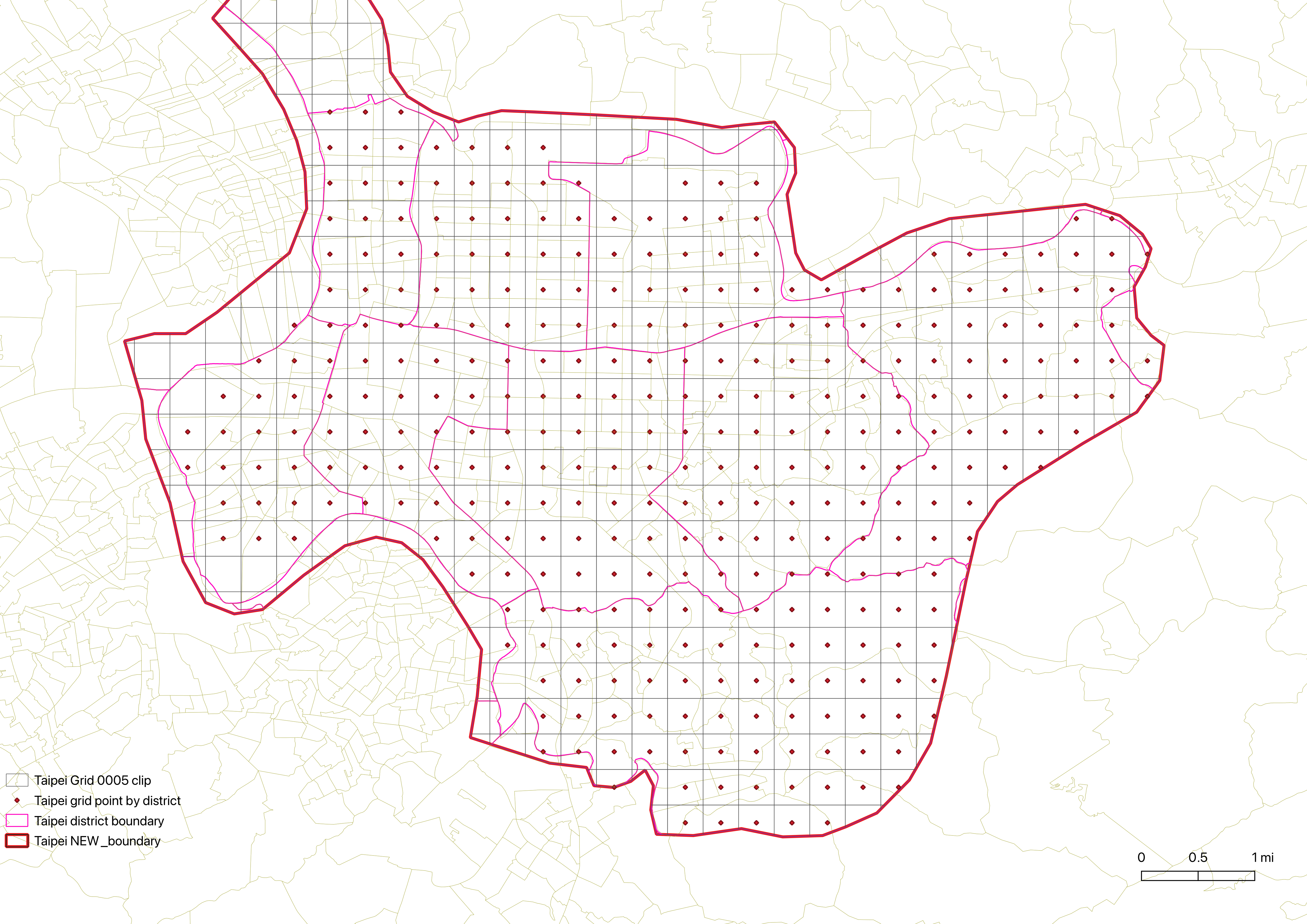

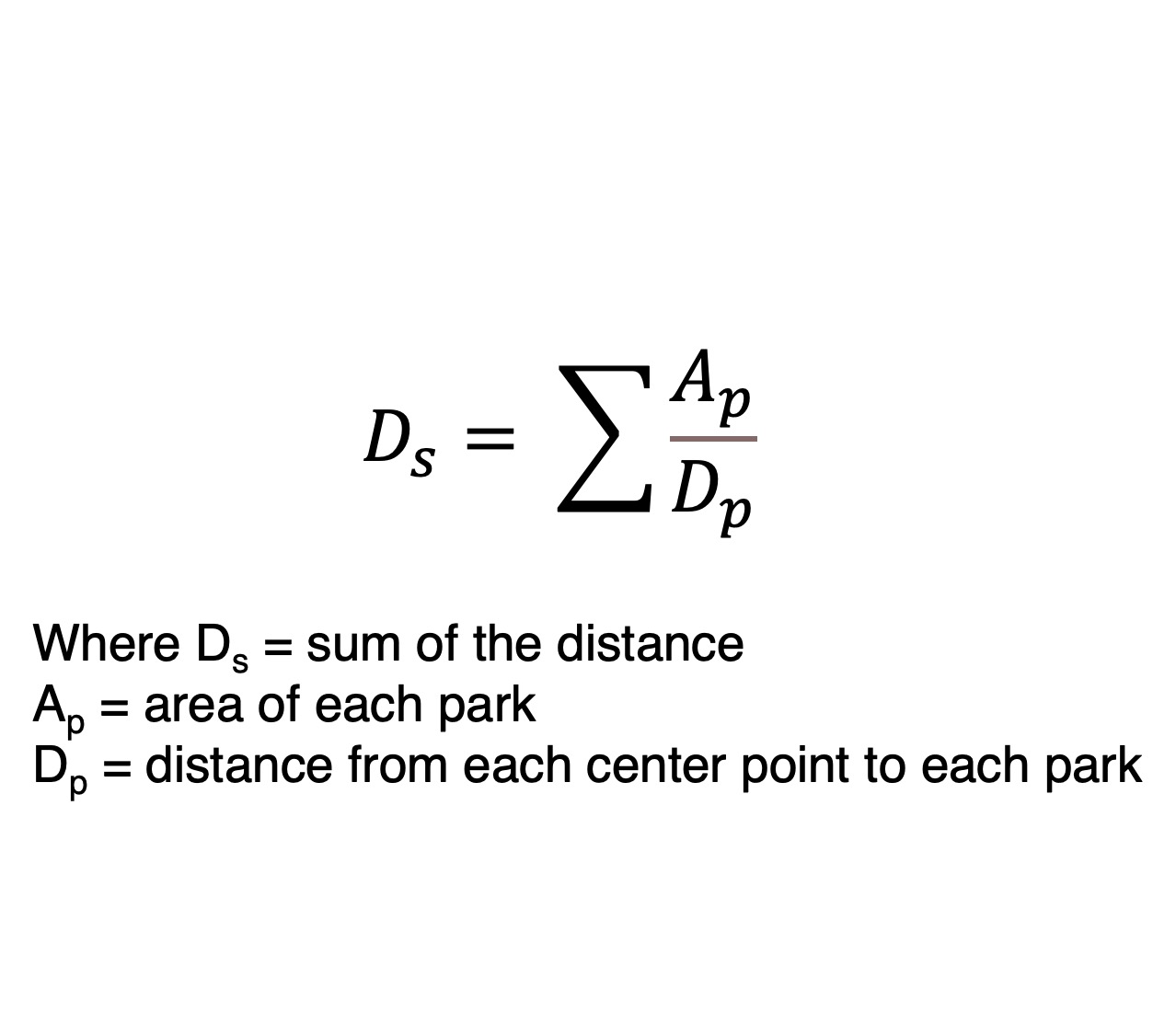
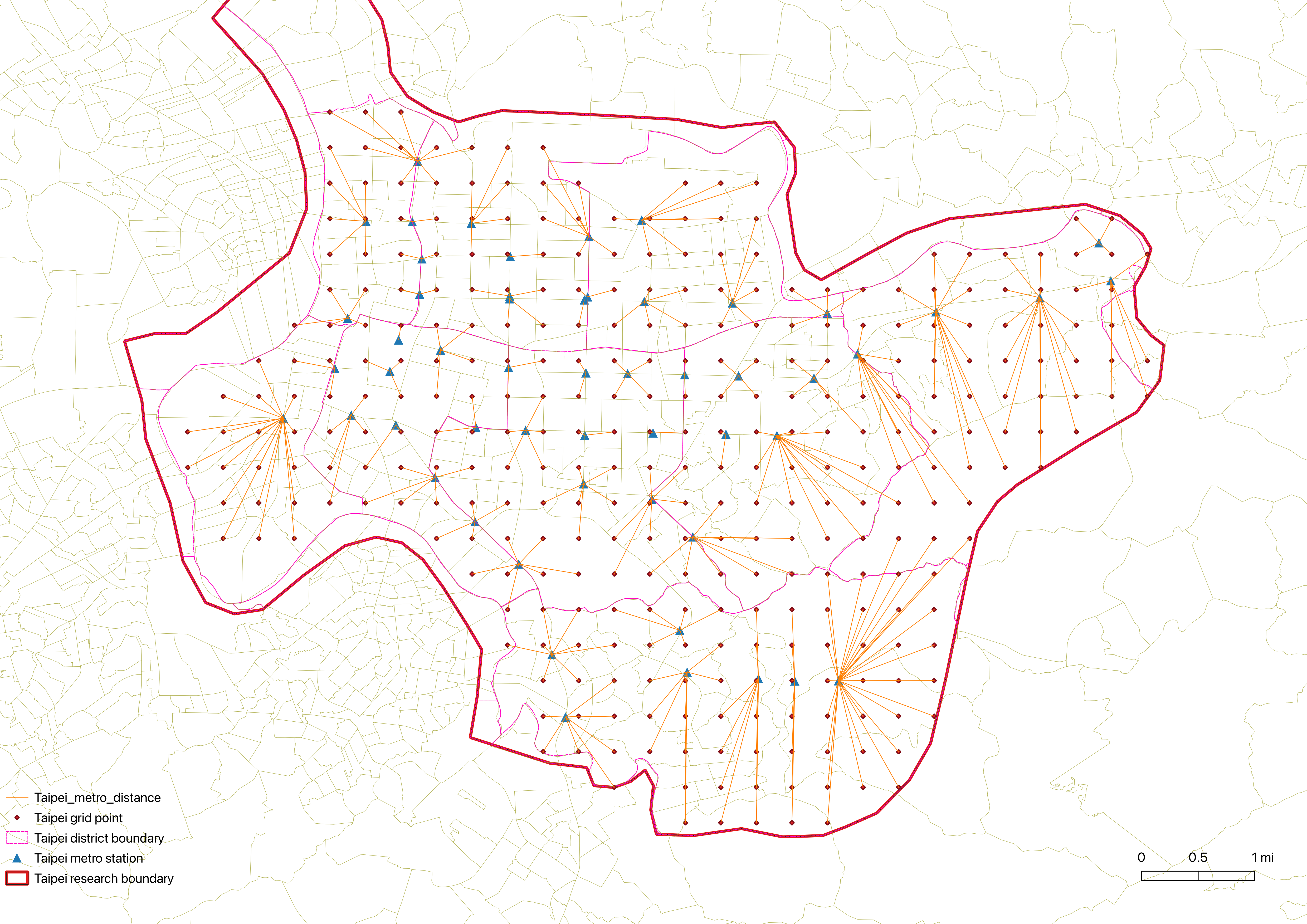
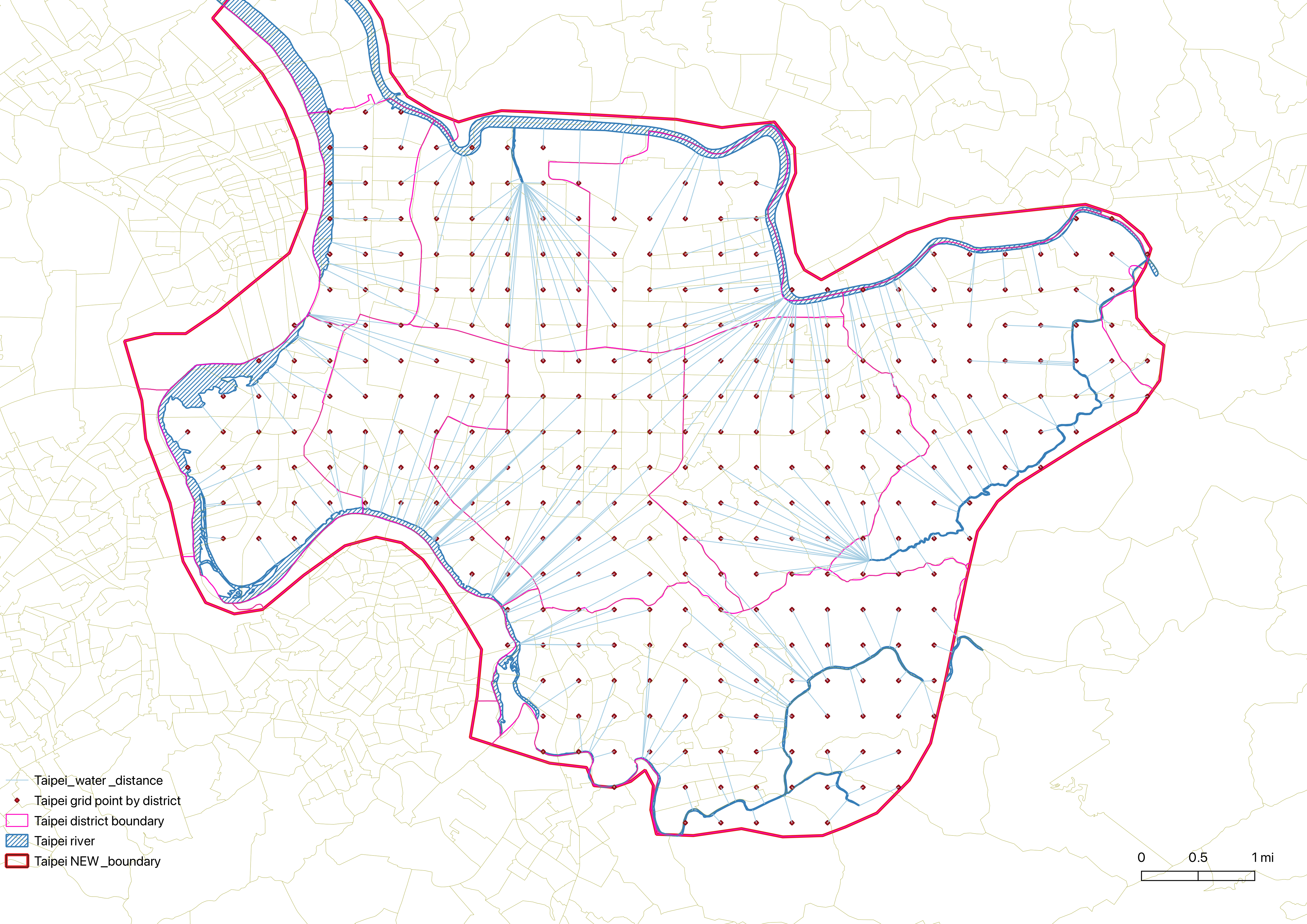
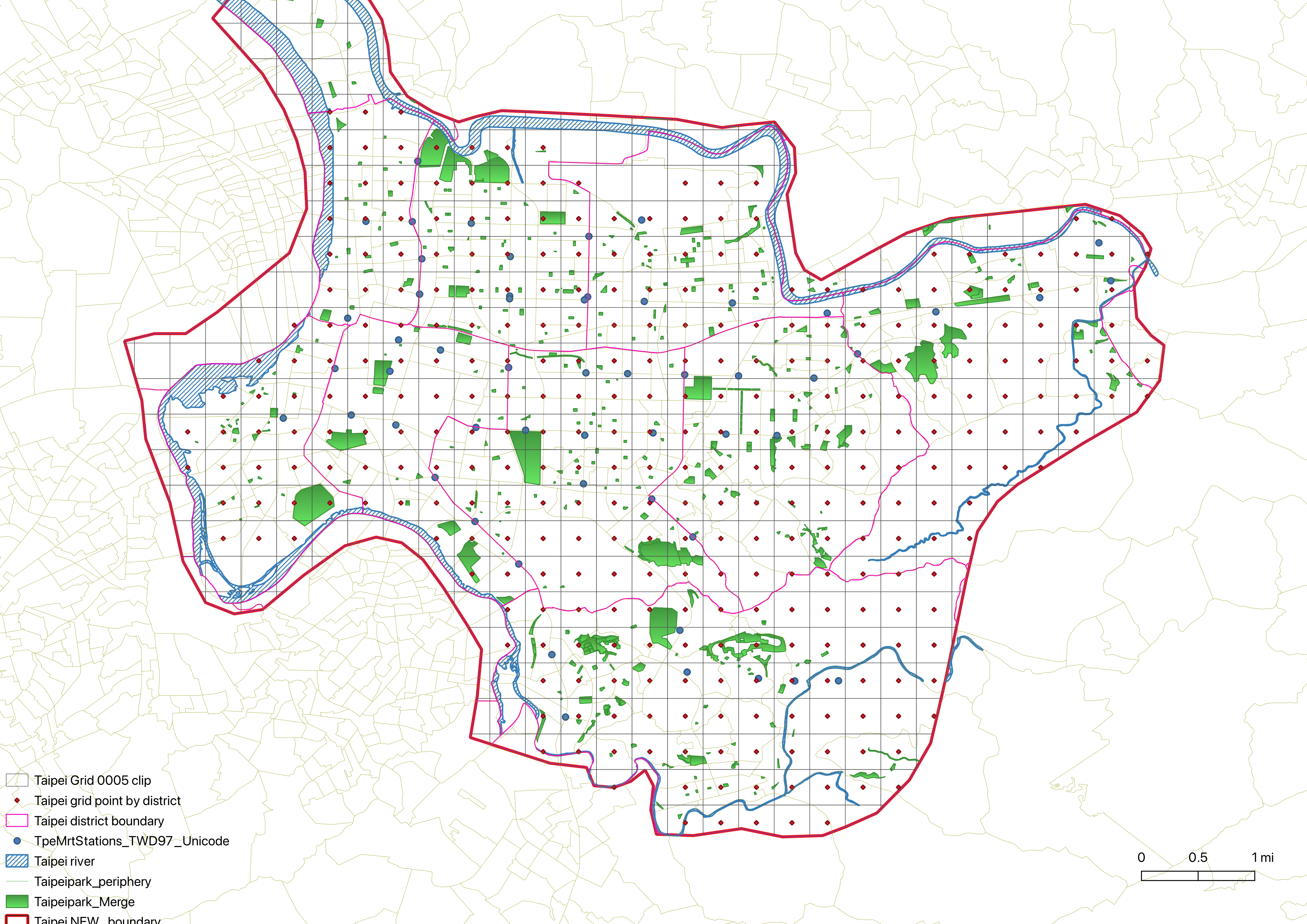
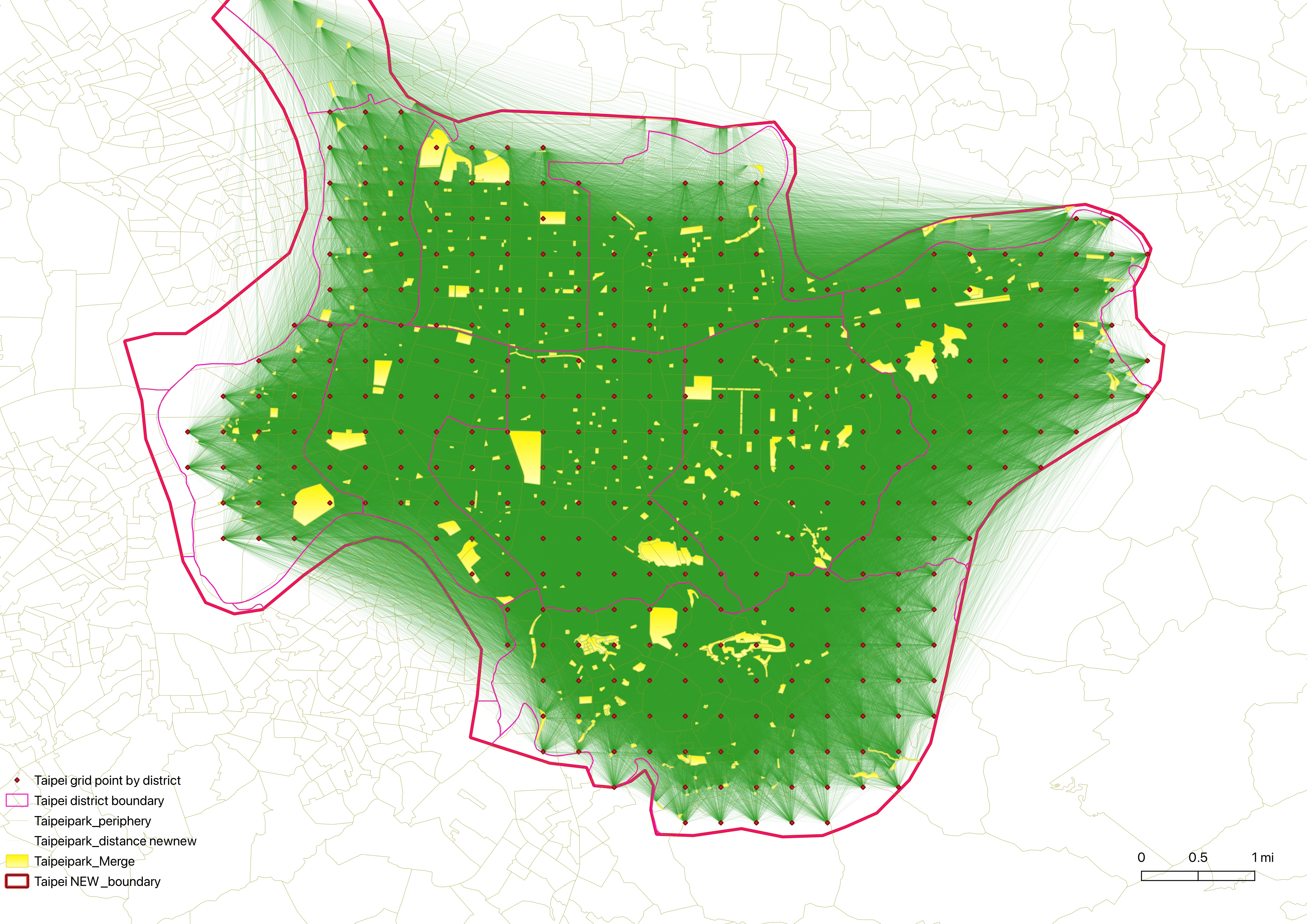
Traditional Real Estate Valuation X Machine Learning Valuation
This project employs both the sales comparison and cost approaches for property valuation. The sales comparison method involves selecting several adjustment factors for sales prices as dictated by the Regulations on Real Estate Appraisal in Taiwan, Taipei’s appraisal guidance, and precedents. These encompass the full array of features and rules utilized in the appraisal process. The cost approach, another traditional valuation method, calculates a property's value by estimating the expenses of constructing a comparable structure anew and then modifying this figure to account for depreciation and obsolescence. The total development cost (TDC) includes land, building, planning and design, advertising, management, and related taxes and fees, with the final valuation being the sum of the land value and new construction cost, less depreciation.
The project utilizes two machine learning models: multiple linear regression and decision tree analysis. These models are adept at capturing non-linear relationships often present in real estate valuation. The decision tree model, while powerful, is adjusted for potential overfitting by trimming outliers based on the interquartile range (IQR) to ensure robust and reliable predictions.
The project utilizes two machine learning models: multiple linear regression and decision tree analysis. These models are adept at capturing non-linear relationships often present in real estate valuation. The decision tree model, while powerful, is adjusted for potential overfitting by trimming outliers based on the interquartile range (IQR) to ensure robust and reliable predictions.
Traditional Real Estate Valuation
Test Data SelectionThe project involves an analysis of three test properties selected across different districts. Each property is designated for residential use, with official sales recorded in 2023 under normal sale conditions, explicitly excluding transactions between relatives. These properties also share a common characteristic in their ownership structure, including land and unit components.
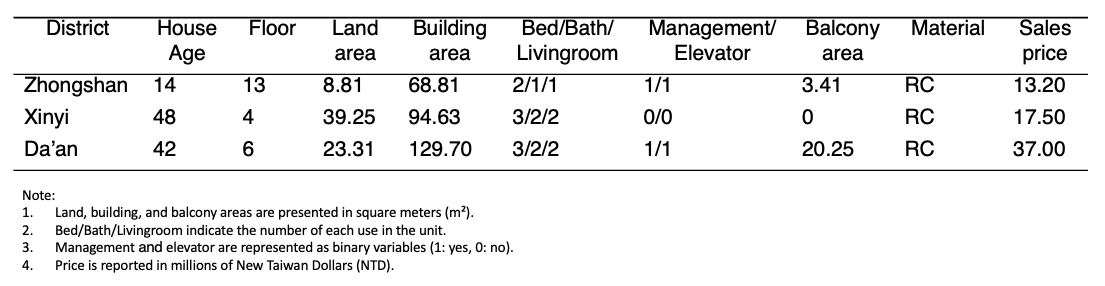
Sales ComparisonThe final assessed value of the test property is determined by averaging three appraised values. Comparable sales are meticulously chosen from the same neighborhood and with similar transaction dates to ensure consistency.
The analysis reveals a significant variance in margins of error, which span from 4.81% in the case of the Xinyi district to 23.45% for the Zhongshan district. These findings indicate that traditional appraisal methods exhibit a high degree of instability and are substantially influenced by the subjective judgment of appraisers.
The analysis reveals a significant variance in margins of error, which span from 4.81% in the case of the Xinyi district to 23.45% for the Zhongshan district. These findings indicate that traditional appraisal methods exhibit a high degree of instability and are substantially influenced by the subjective judgment of appraisers.
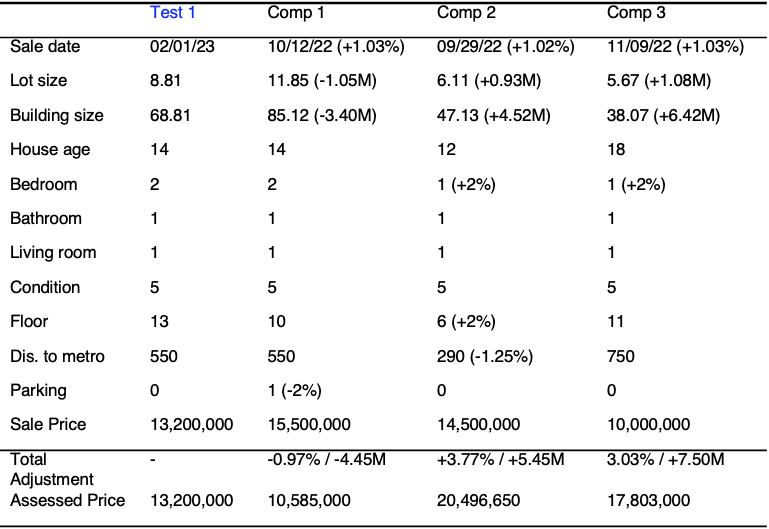
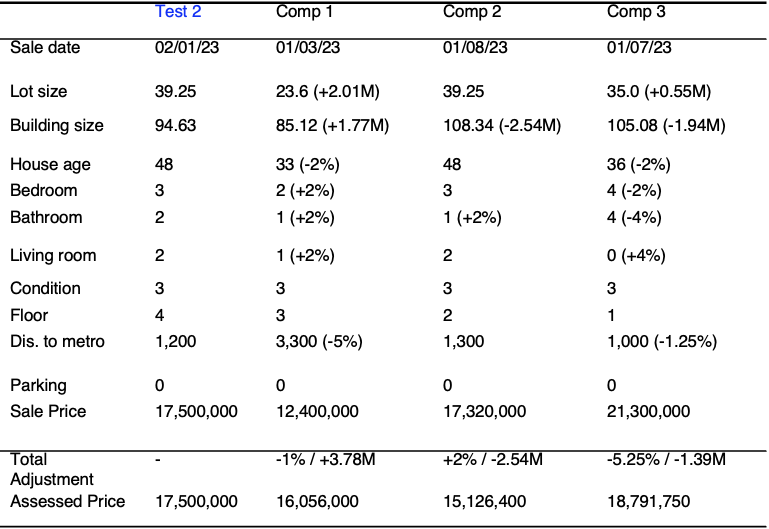
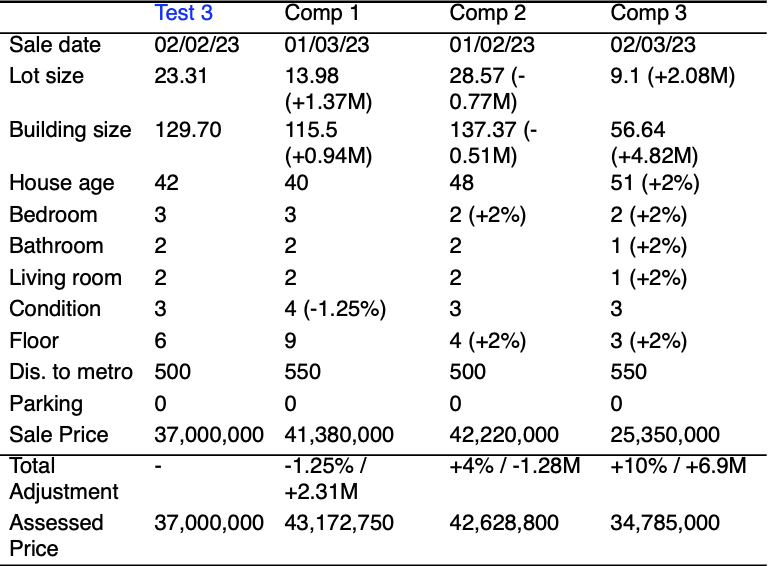
Cost ApproachThe cost approach utilized in the project adheres to specific unit prices for each item and considers the economic life of different building structures. This approach uncovered a pronounced disparity in the margins of error, with a minimal variance of 0.41% in the Zhongshan district and a substantial discrepancy of 41.61% in the Da’an district. Such findings underscore the volatility inherent in traditional valuation methods.
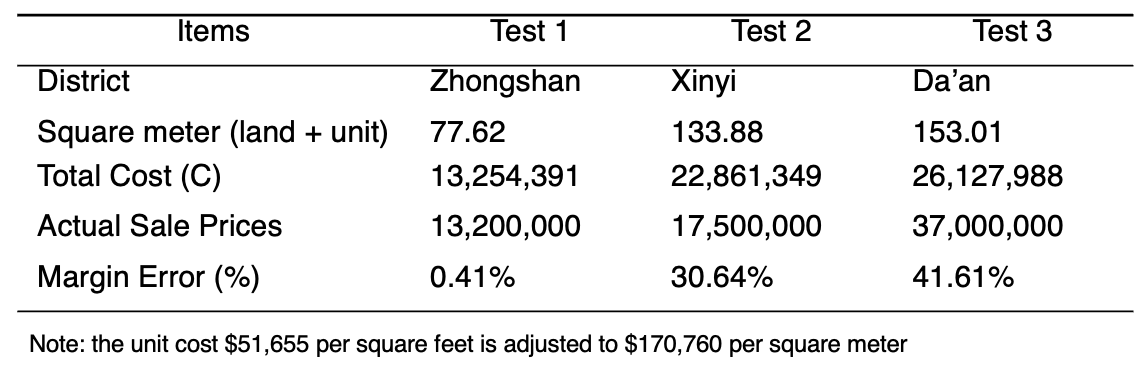
Machine Learning Valuation
Multiple Linear RegressionsModels 1 and 2 are designed to use the Consumer Price Index (CPI) adjusted housing prices as the outcome variable. In contrast, the subsequent models focus on the actual sales prices. Additionally, while Models 1 and 3 solely incorporate Hedonic variables as parameters, Models 2 and 4 integrate sustainability features, resulting in higher R-squared values. This differentiation in model structure underscores the increased predictive power achieved by including sustainability factors.
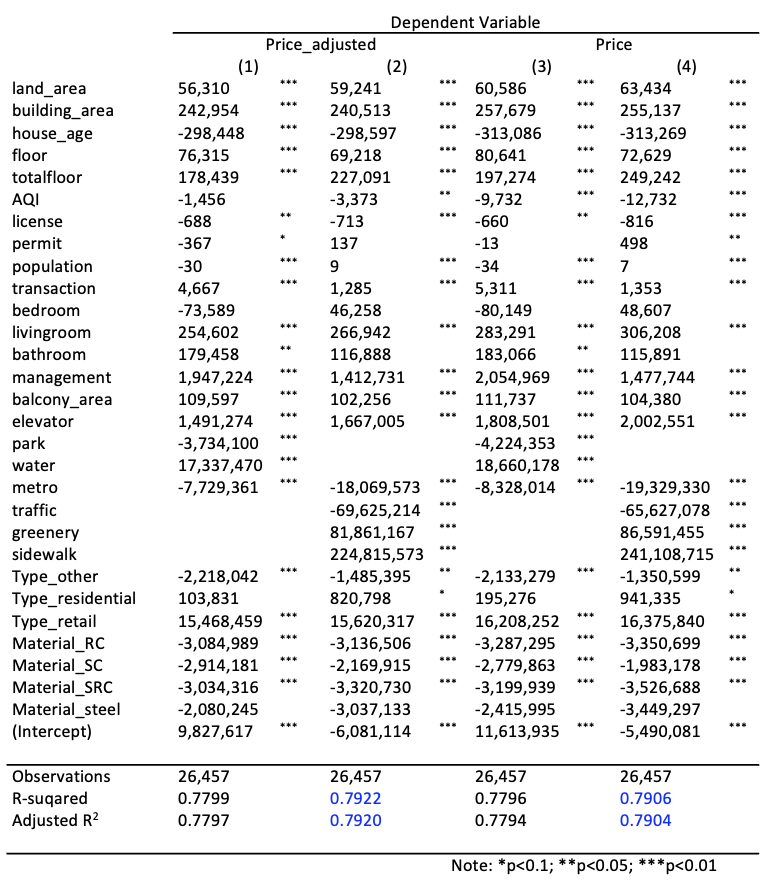
Decision TreesThe project incorporates two distinct decision tree models to refine property valuation. The first model exclusively uses Hedonic features, whereas the second integrates environmental factors. These models reveal that building area and housing age emerge as the most influential factors, while regional population exerts the least impact on property values. Regarding accuracy, the first decision tree exhibited a margin of error of 20.45%. Including environmental features in the second tree led to a marginally improved error rate of 20.39%. Notably, both decision tree models demonstrated lower error margins than those produced by the linear models in the study.
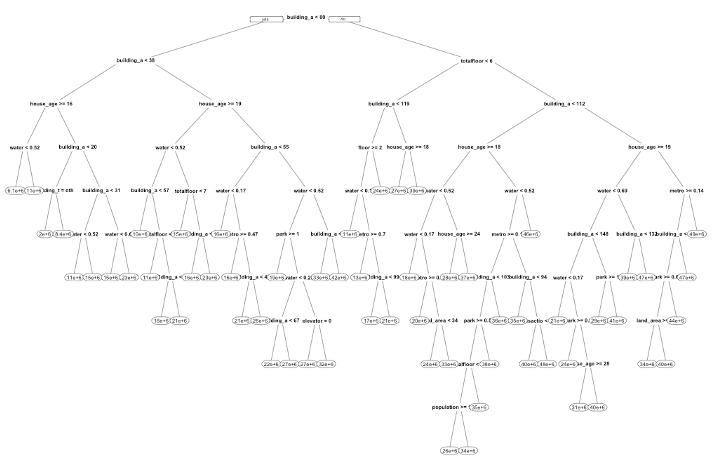
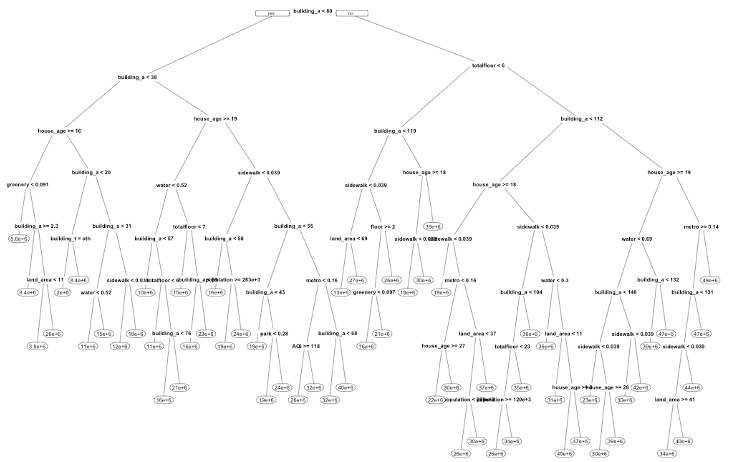
Margins of Errors Across DistrictsAn in-depth analysis of errors across different districts revealed that decision trees exhibit superior predictive power, consistently resulting in the lowest overall errors and error range. This enhanced accuracy is particularly notable when environmental features are incorporated into the model. In comparison, there is room for further refinement in accuracy, and a noteworthy improvement in consistency across various districts has been observed, indicating a promising direction for future enhancements in the valuation process.
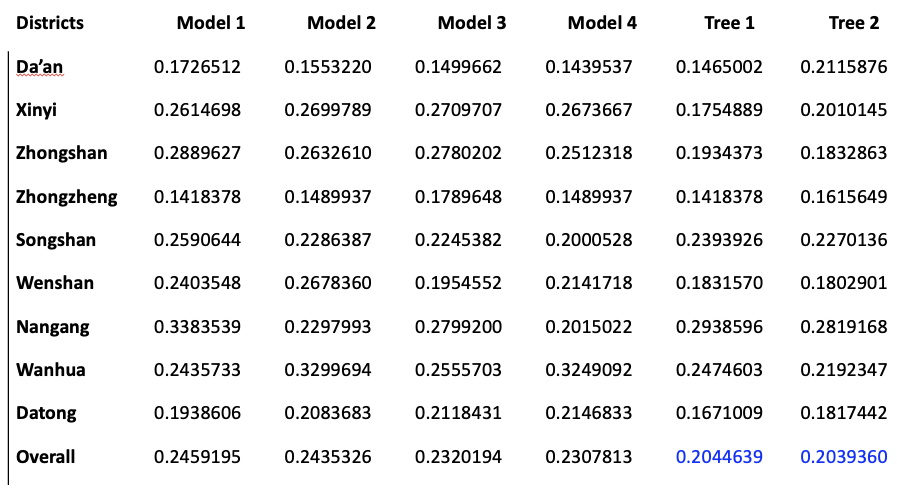
Conclusion & Limitations
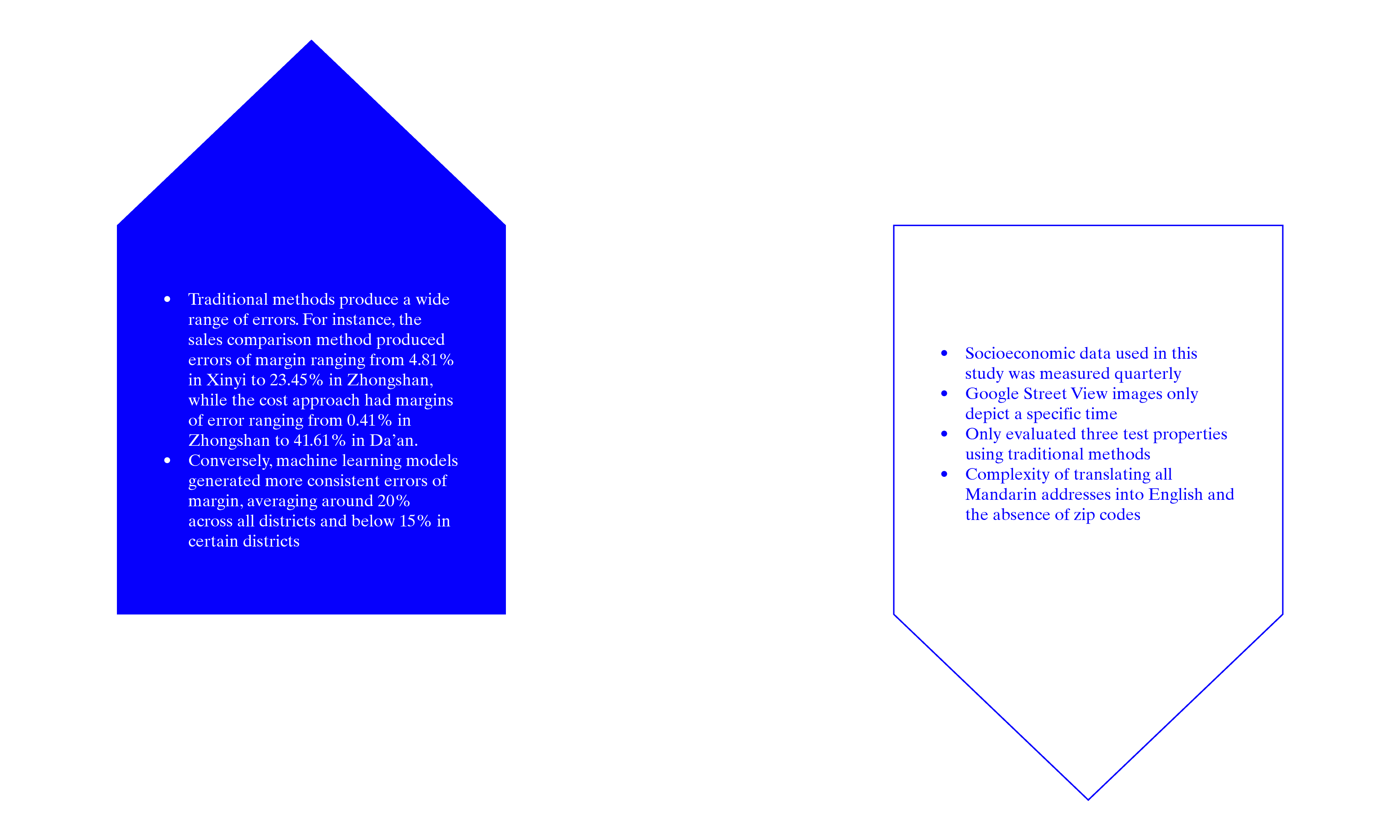
MIT 11.321
Advisor: Fabio Duarte
In collaboration with Hsuan Lo